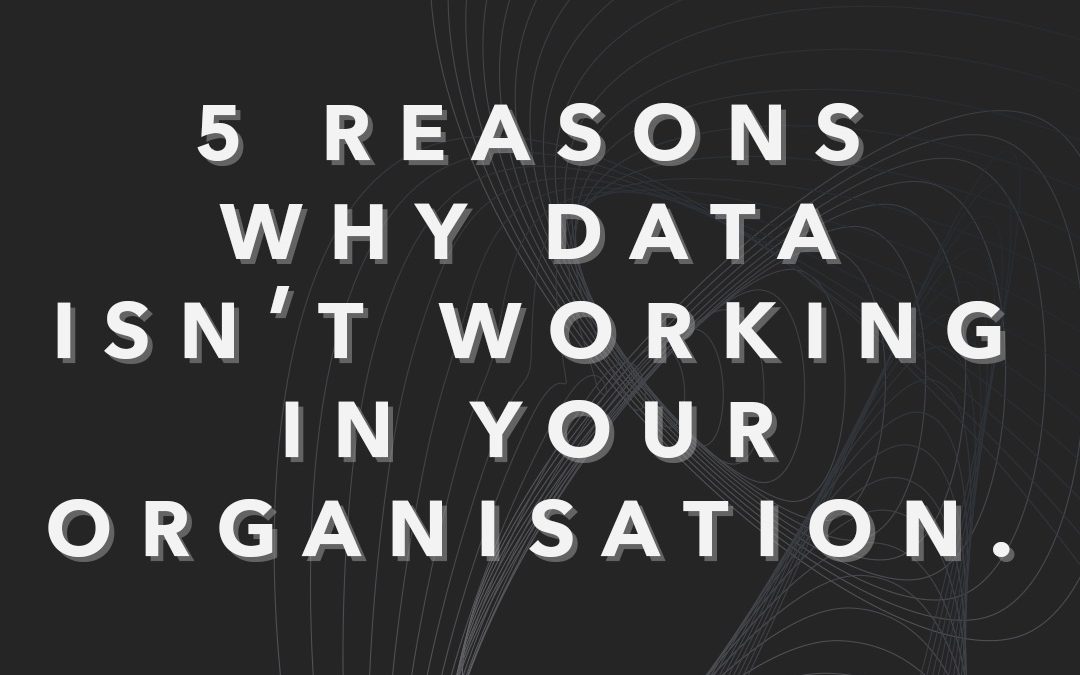
5 Reasons Why Data Isn’t Working in Your Organisation
5 Reasons Why Data Isn’t Working in Your Organisation
The power of data cannot be overstated. We love data and you should too!
Organisations in every industry, everywhere are relying on data to make informed decisions, drive innovation, and gain a competitive edge. And if you’re not… WTF are you doing?
However, despite the potential that data holds, many organizations struggle to harness it effectively.
In this blog post, we’ll explore what data is and how it can be used. After this, highlighting the 5 common reasons why data initiatives may not be working in your organisation and how to overcome these challenges.
Data: What is it? How is it used?
Okay, so what is data? If you didn’t know already (if you already know, skip this bit)
Data refers to raw facts, figures, and statistics that are collected, recorded, or stored in various forms.
It can take the form of numbers, text, images, audio, or any other structured or unstructured information.
Data is the fundamental building block of information and knowledge. It can be categorised into two primary types:
- Structured Data: This type of data is organised into a predefined format and is easy to analyse. It’s often found in databases and spreadsheets, and each data point has a specific meaning. Examples include numerical values in an Excel spreadsheet or customer details in a relational database.
- Unstructured Data: Unstructured data is not organized in a predefined manner. It can be in the form of text, images, audio, or video and doesn’t fit neatly into rows and columns. Examples include social media posts, emails, images, and videos.
Data is a valuable resource that can be harnessed for various purposes in different sectors. Here are some common ways data can be used:
- Decision Making: Data is crucial for informed decision-making. Organizations use data to analyse trends, identify opportunities, and make strategic choices. For example, a retail company may use sales data to decide which products to stock.
- Performance Analysis: Data can be used to assess the performance of processes, products, or individuals. In sports, for instance, performance data is used to evaluate athlete performance and make improvements.
- Predictive Analytics: By analysing historical data, organizations can make predictions about future events. For example, financial institutions use historical transaction data to detect fraudulent activities.
- Personalization: Data is used to tailor experiences for individuals. Online retailers, for instance, use data to suggest products based on a customer’s browsing and purchase history.
- Marketing: Marketers use data to target specific demographics, track campaign performance, and optimize their strategies.
- Customer Insights: Customer data helps businesses understand their customer’s preferences, behaviours, and needs, enabling them to provide better products and services.
- Financial Analysis: Financial institutions rely on data for risk assessment, investment decisions, and fraud detection.
Data can be a powerful tool when collected, processed, and analysed effectively.
However, you might be using data in your organisation. Yet, it is failing or not being used to its fullest potential!
Here are 5 common reasons why may not be working in your organisation and how to overcome these challenges.
Reason 1: Lack of Data Strategy
A clear data strategy is the foundation upon which successful data initiatives are built. Without it, organizations are essentially navigating uncharted waters.
A data strategy encompasses a structured plan for collecting, storing, analysing, and using data to achieve specific business goals. It defines the what, why, and how of data management.
It is basically a business strategy using data.
The consequences of not having a clear data strategy are numerous. It often leads to confusion, redundancy, and a lack of direction. Without a strategy, you may find yourself collecting and storing data that is irrelevant to your business objectives.
To overcome this challenge, look to organisations like Amazon and Google, which have well-defined data strategies. They use data to optimise their operations, enhance customer experiences, and drive growth.
Create a data strategy that aligns with your business goals and ensure it is communicated and followed throughout your organisation.
Understand more about data strategy here.
Reason 2: Data Silos
Data silos occur when different departments or teams in an organisation store data independently, without sharing or integrating it.
This can hinder effective data utilisation, decision-making, and collaboration.
Imagine marketing and sales teams using different data sources, leading to conflicting information and missed opportunities.
To break down data silos, implement systems that allow for easy data sharing and integration. Encourage cross-functional collaboration and ensure that data is accessible to all who need it.
Tools like data warehouses and collaboration platforms can be instrumental in this process.
Understand more about Data Silos and how to eliminate them here.
Reason 3: Inadequate Data Quality
Poor data quality can be a major roadblock to effective data utilisation. Have you heard the term s#!t in = s#!t out??
Inaccurate, incomplete, or outdated data can lead to misguided decisions and analysis. To mitigate these risks, organisations must prioritise data quality.
Start by implementing data validation and cleansing processes. Regularly audit your data for accuracy and completeness. Establish data quality standards and make sure they are consistently upheld across the organization.
Reason 4: Resistance to Change
Implementing data-driven practices often encounters resistance from employees or teams comfortable with existing methods.
It’s essential to recognize that data-driven decision-making may necessitate changes in established workflows and practices.
To address resistance, consider offering training and education to your employees. Show them the benefits of data-driven decision-making through success stories from other organisations.
Create a supportive culture where employees are encouraged to embrace data and innovation.
Also, with data automation your team’s output will increase and reduce costs – it is obviously the way forward. Make your team efficient and happy!
Reason 5: Insufficient Data Governance
Data governance is the framework that ensures data is managed, utilised, and protected effectively within an organisation.
Without robust data governance, data-related issues can easily spiral out of control.
To establish effective data governance, define roles and responsibilities for data management, set data access controls, and enforce data policies and standards.
This will ensure that data is protected and used ethically and responsibly.
Conclusion
In conclusion, data is an invaluable asset for any organisation, but to unlock its full potential, it’s crucial to address common bottlenecks that may hinder its effectiveness.
By implementing a clear data strategy, breaking down data silos, ensuring data quality, addressing resistance to change, and establishing strong data governance, you can transform your organization into a data-driven powerhouse.
Obviously, these aren’t the ONLY reasons why working with data isn’t working within your organisation. But these are the most common reasons why!
Stick around though, we may go more in-depth into your specific reasons soon as to why data isn’t working in your organisation!
Take a moment to assess your organisation’s data practices.
Are any of the challenges mentioned in this blog post affecting your data initiatives? If so, consider taking steps to address them and unlock the full potential of data in your organisation.
Fill out the form below to reach out if you have questions or need further guidance on any of these topics!
Let’s make data WORK! You need it to have a thriving business in 2024!
By submitting your information, you’re giving us permission to email you. You can unsubscribe at any time. FYI: We won’t spam your inbox!