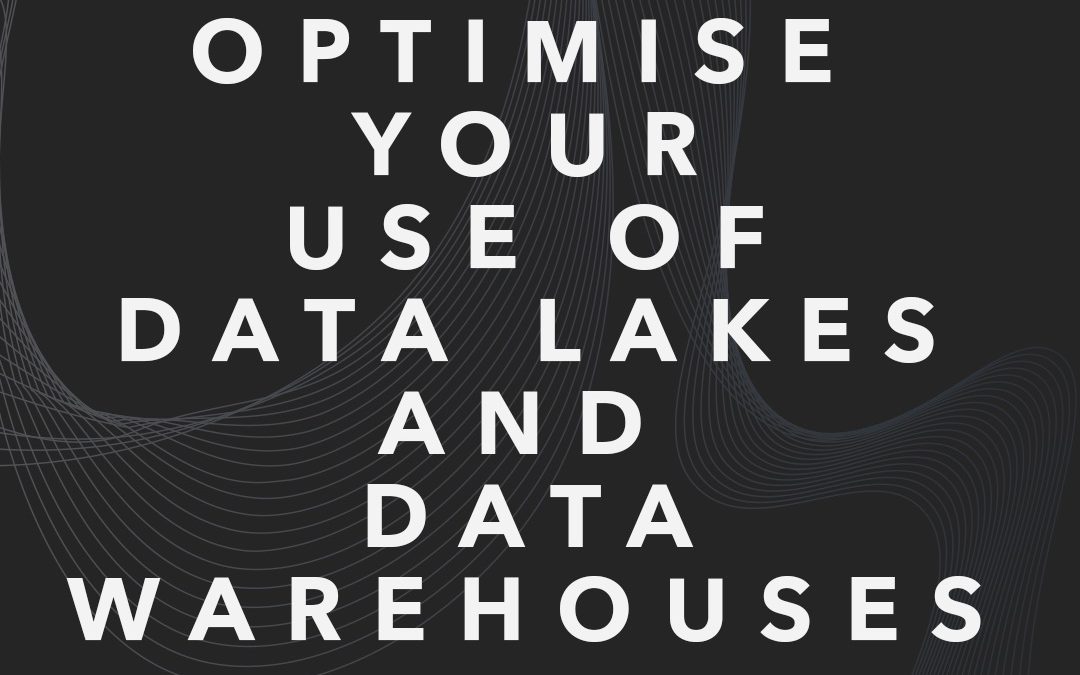
Optimise Your Use of Data Lakes and Data Warehouses
Optimise Your Use of Data Lakes and Data Warehouses
Managing vast amounts of information efficiently is crucial.
Optimising your data lake or data warehouse can lead to significant improvements in performance and cost savings.
But beyond these benefits, there is a growing need for corporate social responsibility (CSR) in IT operations. This means adopting practices that not only enhance efficiency but also reduce environmental impact.
This blog post explores how you can optimise your data solutions with CSR in mind, ensuring energy-efficient and sustainable data management.
Importance of Corporate Social Responsibility in Data Solutions
Corporate social responsibility in data management involves adopting practices that reduce environmental impact and promote sustainability.
As businesses grow increasingly reliant on data, the energy consumption of data centers and storage solutions has become a significant concern. By integrating CSR principles into your data management strategy, you not only contribute to environmental preservation but also improve your company’s reputation and operational efficiency.
Sustainable practices can lead to cost savings, enhance regulatory compliance, and build trust with stakeholders.
Strategies for Energy-Efficient Data Management
Reducing Energy Consumption in Data Storage
Energy consumption in data storage can be minimised through various methods.
Virtualisation helps consolidate workloads, reducing the need for physical servers and thus cutting down on energy usage.
Implementing cooling system optimisation and advanced power management techniques further enhances energy efficiency.
When purchasing hardware, consider the power consumption relative to performance.
Opt for solid-state storage, which is not only faster but also more energy-efficient compared to traditional hard drives.
Reducing network latency by strategically placing data closer to computer resources can also lower energy costs and improve processing speed.
Optimising Data Processing Practices
Data processing is a major contributor to energy consumption in IT operations. Adopting efficient processing practices can significantly reduce this impact.
Regularly review and refine your data processing workflows to eliminate unnecessary steps and optimise performance.
Implementing best practices such as data compression, deduplication, and efficient indexing can reduce the volume of data processed and stored, leading to energy savings.
Additionally, training your IT team to follow energy-efficient guidelines can help ensure that sustainability is a core aspect of data management strategy.
Choosing Green Cloud Services
Cloud services offer a scalable solution for data storage and processing, but they also consume significant energy.
When selecting a cloud provider, consider their commitment to green energy and sustainability. Look for providers that utilise renewable energy sources and have robust environmental policies. Assess their data centre efficiency metrics, such as Power Usage Effectiveness (PUE), to ensure they operate in an energy-efficient manner.
Choosing a green cloud service not only reduces your company’s carbon footprint but also supports the broader transition to sustainable energy practices in the tech industry.
Best Practices for Minimising Data Duplication
Data duplication is a common issue that leads to increased storage requirements and energy consumption.
Implementing best practices to minimise data duplication can enhance efficiency and sustainability.
Conduct regular audits to identify and eliminate redundant data.
Utilise data deduplication technologies that automatically detect and remove duplicates across your storage systems.
Additionally, consider adopting snapshot management techniques that reduce the need for full data backups, thereby saving storage space and energy.
By streamlining your data storage, you can achieve significant energy and cost savings.
Conclusion
Optimising your data lake or data warehouse with a focus on corporate social responsibility is not only beneficial for the environment but also for your business.
By reducing energy consumption, implementing sustainable practices, and choosing green cloud services, you can enhance the efficiency and sustainability of your data management operations.
These strategies contribute to cost savings, improved performance, and a positive corporate image.
Embrace these practices to ensure your data solutions are both efficient and environmentally responsible.
Schedule a Call Now.
Ready to optimise your data lake or data warehouse for maximum efficiency and sustainability?
Let’s take the next step together.
Schedule a call with our experts today to discover how you can implement energy-efficient practices and enhance your corporate social responsibility. Our team will provide personalized insights and solutions tailored to your business needs.