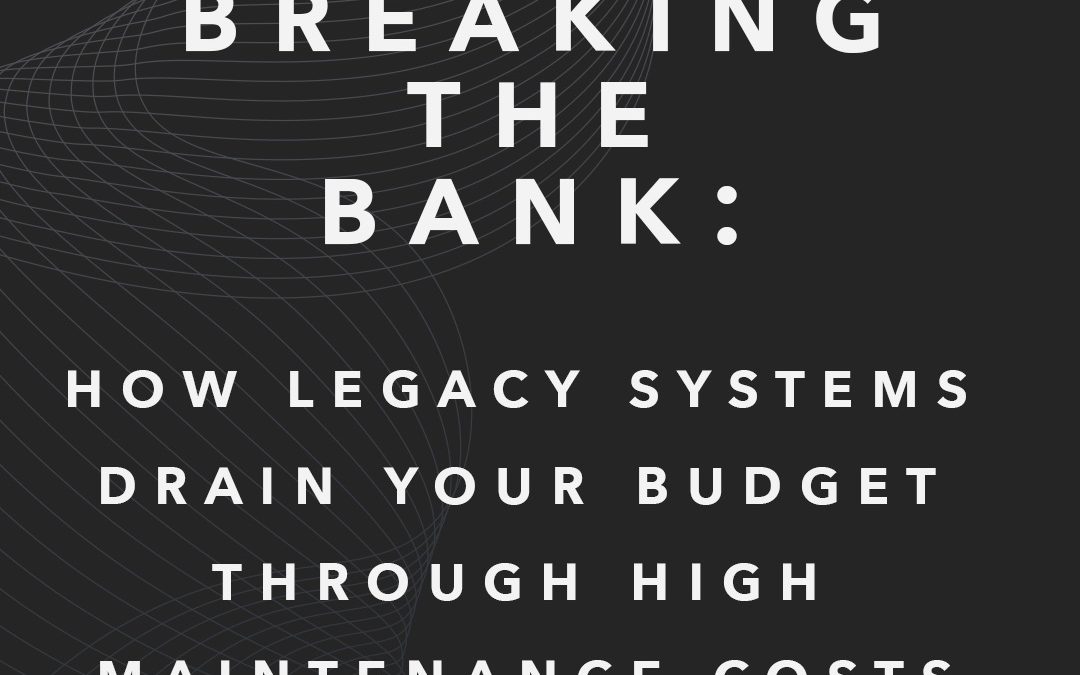
Breaking the Bank: How Legacy Systems Drain Your Budget Through High Maintenance Costs
Breaking the Bank: How Legacy Systems Drain Your Budget Through High Maintenance Costs
Picture this: a thriving business, built on years of hard work and dedication, suddenly finds itself shackled by the chains of outdated technology. Despite its past successes, the organisation is now faced with a daunting reality – a relentless drain on its financial resources.
In the ever-evolving landscape of technology, the burden of legacy systems has become a silent but powerful force, quietly draining away funds that could otherwise fuel innovation and growth.
Consider this startling statistic that highlights the pervasive impact of legacy systems: according to recent studies, over 80% of businesses still grapple with challenges posed by legacy technology. This is not a mere inconvenience; it’s a financial swamp that organisations of all sizes find themselves sinking into, and the consequences are far-reaching.
Legacy systems are the remaining of outdated, software, hardware and infrastructure that were once the backbone of operational processes. These systems, although once cutting-edge, now hinder an organisation’s ability to adapt and respond to the demands of the modern business environment.
Whether it’s archaic software applications, obsolete hardware or systems lacking compatibility with contemporary tools, legacy dependencies have permeated business. The prevalence of these outdated systems is not a testament to their effectiveness but rather a reflection of the reluctance or challenges organisations face in breaking free from the past.
The reliance on systems that have the potential to break the bank, places business at a perilous crossroads where financial stability is compromised by the ever-increasing burden of maintenance costs.
The Rising Tide of Legacy Maintenance Costs
To comprehend the full scope of the financial challenge posed by legacy systems, it’s crucial to dissect the traditional lifecycle costs that accompany their presence.
Legacy systems, but their very nature, demand ongoing maintenance to remain operational. This includes routine tasks such as updates, patches, and troubleshooting. Yet as these systems age, the costs associated with sustaining them tend to follow an upward trajectory.
From the initial implementation to the ongoing support required for outdated software and hardware. By understanding the layers of expenditure involved, we can better grasp why the seemingly static presence of legacy systems can be a ticking financial time bomb.
The Hidden Costs Unveiled
Beyond the visible surface of routine maintenance lies a realm of hidden costs that often escape the scrutiny of businesses. Let’s dive deep into these obscured financial intricacies exposing the often-overlooked expenses tied to the ongoing maintenance of legacy systems.
From licencing fees and software updates to compatibility challenges, these are some layers of expenditure that silently accumulate, contributing to the financial strain on organisations.
By bringing these hidden costs into focus, businesses can gain a comprehensive understanding of the financial implications associated with clinging to outdated technology.
This financial strain, while apparent on balance sheets, extends beyond mere numbers, impacting the organisation’s ability to allocate budgets for strategic initiatives and technological advancements.
Loss of Competitive Edge:
Hidden Cost: Inability to adopt cutting-edge technologies and practices due to budget constraints on maintenance.
Impact: Competitors who invest in modern systems may outpace the organisation, leading to a loss of market share and revenue.
Reduced Employee Morale and Turnover:
Hidden Cost: Frustration and burnout among employees dealing with outdated systems daily.
Impact: Decreased job satisfaction increased turnover rates, and the subsequent costs of hiring and training new staff.
Customer Dissatisfaction and Attrition:
Hidden Cost: Customer dissatisfaction resulting from service delays, errors, or outdated features.
Impact: Attrition of customers to competitors with more agile and technologically advanced offerings, leading to revenue loss.
Increased Risk of Data Breaches:
Hidden Cost: Security vulnerabilities in outdated systems may result in a higher risk of data breaches.
Impact: Financial fallout from managing and mitigating the aftermath of a breach, including legal fees, regulatory fines, and damage to the organisation’s reputation.
Inefficiencies in Business Processes:
Hidden Cost: Inefficient workflows and operational bottlenecks due to outdated system limitations.
Impact: Increased labour costs as employees spend more time navigating workarounds, leading to decreased overall operational efficiency.
Lack of Scalability:
Hidden Cost: Inability to scale operations efficiently due to rigid legacy systems.
Impact: Missed opportunities for growth and expansion, with potential revenue streams left untapped.
Extended Downtime and Disruptions:
Hidden Cost: Unplanned downtime and disruptions during maintenance activities.
Impact: Loss of revenue during downtime, potential penalties for failing to meet service level agreements, and damage to customer trust.
Vendor Lock-In:
Hidden Cost: Dependence on legacy vendors for support and updates.
Impact: Limited negotiating power and potential price hikes from vendors, leading to increased overall costs.
Regulatory Non-Compliance:
Hidden Cost: Challenges in adapting legacy systems to meet evolving regulatory requirements.
Impact: Fines and legal consequences for non-compliance, as well as the cost of retrofitting systems to meet new regulations.
Obsolescence Risks:
Hidden Cost: The risk of key components of legacy systems becoming obsolete and difficult to replace.
Impact: Increased costs associated with finding or developing alternatives, potentially requiring a major system overhaul.
All these represent a nuance in hidden costs that organisations must carefully consider when evaluating the impact of legacy systems maintenance on their overall financial health and operational efficiency.
Recognising and addressing these hidden costs becomes imperative for organisations seeking to break free from the constraints of outdated technology and pave the way for strategic growth and resilience.
Calculating the True Financial Impact
Maintenance costs, often perceived as static, can have a compounding effect over time. The trajectory of increasing maintenance costs illustrates how seemingly modest expenses escalate into significant financial burdens. By projecting these costs over the extended lifespan of legacy systems, organisations can gain insight into the cumulative financial strain imposed on their budgets.
Through visualising the compounding effect, businesses can make informed decisions about the sustainability of relying on outdated technology.
While the direct financial impact of legacy maintenance is crucial, understanding the opportunity costs is equally imperative. The indirect costs are incurred when budgets are allocated to legacy maintenance at the expense of innovation. Organisations often find themselves at a crossroads where resources dedicated to maintaining outdated systems could be redirected towards strategic initiatives and technological advancements. By assessing these opportunity costs, businesses can weigh the value of investing in modernisation efforts against the perpetuation of legacy dependencies.
By quantifying the true financial impact, considering the compounding nature of maintenance costs, and addressing opportunity costs, businesses can chart a course toward financial resilience and strategic growth. The journey to break free from the financial shackles of legacy systems begins with a clear understanding of the multifaceted impact on your organisation’s fiscal health.
Escaping the Vicious Cycle: Strategies for Modernisation
By exploring the concept that modernisation is not merely an expenditure by an investment. Modernisation is a long-term cost saving.
Upgrading technology infrastructure, while requiring an initial commitment, serves as a safeguard against the escalating maintenance costs associated with legacy systems. Modernisation is a forward-looking financial strategy, as well as optimising your overall organisation, you can understand how a well-timed investment can break the vicious cycle of perpetual maintenance expenses that are nuanced with legacy systems.
Transitioning away from legacy systems is not just about mitigating ongoing maintenance costs; it’s an investment in future returns.
The potential return on investment that organizations can realize by migrating away from legacy systems. By assessing the gains in operational efficiency, improved productivity, and enhanced adaptability to market changes, businesses can quantify the financial benefits of modernization. Understanding the potential returns becomes a pivotal factor in justifying the decision to break free from the constraints of outdated technology.
The journey towards modernisation is multifaceted, and you must tailor your approach to your unique needs and constraints.
Whether the modernisation is a gradual phased transition, a comprehensive system overhaul, or embracing emerging technologies, like cloud solutions, each strategy comes with its own set of costs and benefits.
As you navigate through these strategies, the overarching goal is to empower organisations with the knowledge needed to break free from the vicious cycle of legacy system challenges.
By embracing modernisation as a strategic financial move, understanding the potential returns, and exploring diverse modernisation strategies, businesses can pave the way for a resilient, technologically advanced future.
The key is not just in escaping the past, but in crafting a pathway towards sustained innovation and financial well-being.
Conclusion
In this exploration of the financial intricacies surrounding legacy system maintenance, it is evident that the detrimental impact on budgets goes beyond the surface.
The compounding maintenance costs, often underestimated and hidden, cast a shadow over organizational finances, hindering growth and innovation.
To recap, legacy system maintenance costs create a pervasive financial strain, draining resources that could be channelled into strategic initiatives. From hidden operational inefficiencies to the escalating burden on staff productivity, the true cost extends beyond the dollars on balance sheets. The financial toll becomes a weighty impediment, limiting budgets for critical technological advancements and stifling an organization’s ability to stay competitive in a rapidly evolving landscape.
The urgency for businesses to break free from the chains of legacy systems cannot be overstated. The cost of maintaining outdated technology is a relentless cycle that perpetuates budgetary constraints and operational inefficiencies. The longer your organisation hesitates to break free, the more entrenched it becomes in a financial swamp.
This is a call to action, urging businesses to recognise the need for change and embrace a future unburdened by the weight of legacy dependencies.
In closing, the path to financial stability lies in a forward-thinking approach to technology investment. By viewing modernisation not as a mere expense but as a strategic investment, organisations can secure long-term financial stability.
Embracing cutting-edge technologies, exploring innovative solutions, and adopting a proactive stance toward change can free businesses from the shackles of perpetual maintenance costs. It’s a journey toward resilience, adaptability, and a future where technology empowers rather than hinders.
As businesses navigate the evolving landscape of technology, the imperative is clear: break free from the past, embrace modernisation, and secure a financial future that is agile, innovative, and ready to thrive in the face of technological evolution.
Ready to break free from the financial shackles of legacy systems and pave the way for a more resilient future?
Download our comprehensive whitepaper now to gain invaluable insights into the true cost of maintaining outdated technology and the strategic advantages of modernisation and more
Don’t let outdated technology impede your financial stability. It’s time to act, innovate, and secure a future where your organization thrives in the face of technological evolution.
Download our whitepaper today and embark on the path to financial resilience and technological empowerment.
The journey towards financial liberation begins with the courage to step out of the shadow of legacy systems and into the light of a technologically advanced future.
FREE DOWNLOAD: From Legacy to Leading Edge: Transformative Strategies for CIOs, CDOs, and CTOs in Investment Management
By submitting your information, you’re giving us permission to email you. You can unsubscribe at any time. FYI: We won’t spam your inbox!